The current consensus is that the Modern Data Stack has four layers, five if you count observability. Each plays an important part, building on each other towards the dream of accurate, reliable analytics that can help businesses increase revenue and save on costs by becoming more intelligent. So, what’s the problem?
It’s not so much a problem as it is a fundamental limitation. This human-driven pipeline means it does not matter how powerful your data warehouse is when the real constraining factor to getting actionable insights is human manual labor – and by that I mean how fast a data analyst can write a query.
If it’s just one query – sure, that’s doable. Two queries? Alright, we got this. What about when there are multiple investigations necessary because we need to figure out 1. Why we lost customers in April of this year, and 2. Why the website keeps crashing on Thursdays (that the product team needs yesterday), 3. The financial summary report of last month, and 4. The customer success team has a few questions about company and product IDs but it’ll be just a few quick questions this time (they promise!)
Sound familiar?
So, what is this 6th layer about and how can it help with this situation? NLP (natural language processing) technology utilizing generative AI (while the term has blown up, it really just means large deep learning models that achieve state-of-the-art performance at natural language processing) is starting to remove the limitations that organizations face due to the human-driven pipeline. It offers the ability to integrate into your own data stack, and generate SQL answers in real-time to questions asked in natural language.
Integrating generative AI as a padded layer between BI users and the data, allows analysts to continue working on their big investigations while BI users can ask the data themselves, in natural language and without any specific training on coding jargon or SQL knowledge. This removes the necessity of waiting on an analyst to have time, and allows BI users to get their answers much quicker.
So, how can we close the loop from data to business user? We can start by setting a strategic priority to invest in modernizing data infrastructure in a way that strengthens agility in mining actionable insights. This is where generative AI offers a unique advantage to growth-minded businesses.
Generative AI and the Future of the Modern Data Stack
A colleague of mine made an analogy between the modern data stack and a 5-layer bean dip at a party. Sure, you could eat it straight up, but you’d be missing out on the real enjoyment you would get out of the dip if you just had a better utensil. Enter: the tortilla chip. Like a tortilla chip, generative AI cuts through the 5 layers and turns your data into a bite-sized snack for everyone at the party to enjoy.
Metaphor aside, think of generative AI as an intelligent layer between your BI and warehousing layers, closing the loop between business needs, the data team and the business user.
In the conversation around democratization, generative AI can automate the way past most top-of-mind concerns like ownership, downtime, demonstrating platform ROI and creating a data culture throughout your organization. It’s a solution amenable to all because it hands the querying process back to business users.
AI won’t solve every problem for your data team. It does, however, provide irreplaceable utility by connecting your company’s non-technical users to the actionable insights they urgently need hidden in piles of raw data.
Like any solution, generative AI’s power has limitations and pitfalls. For starters, it’s only as good as the data it’s trained on, and it cannot fix your data quality (yet). On the human side, as we’re all collectively learning, weaning business users who have traditionally been dependent on analysts of it will be a matter of behavioral and organizational change.
Still, with many teams reporting that they spend up to half of their time acting as a service organization to external stakeholders instead of finding insights that grow business, the problem to solve, perhaps one of the most pertinent problems since generative AI became available, is to break the bottleneck between backlogged analysts and business users.
Using natural language processing, AI allows users to query data directly using their natural speech patterns. Where SQL was once required, now even a non-technical employee from customer success to operations, and everyone between can ask the warehouse for what they need, without any additional training required.
Integrating generative AI as a padded layer between BI users and the data, allows analysts to continue working on their big investigations while BI users can ask the data themselves, in natural language and without any specific training on coding jargon or SQL knowledge. This removes the necessity of waiting on an analyst to have time, and allows BI users to get their answers much quicker.
You’ve made all of these investments–emotional and financial–in creating a world-class data stack. Now hand the ownership of the querying process back to those business users, realizing the business value-focused promise of data, closing the loop for your company.
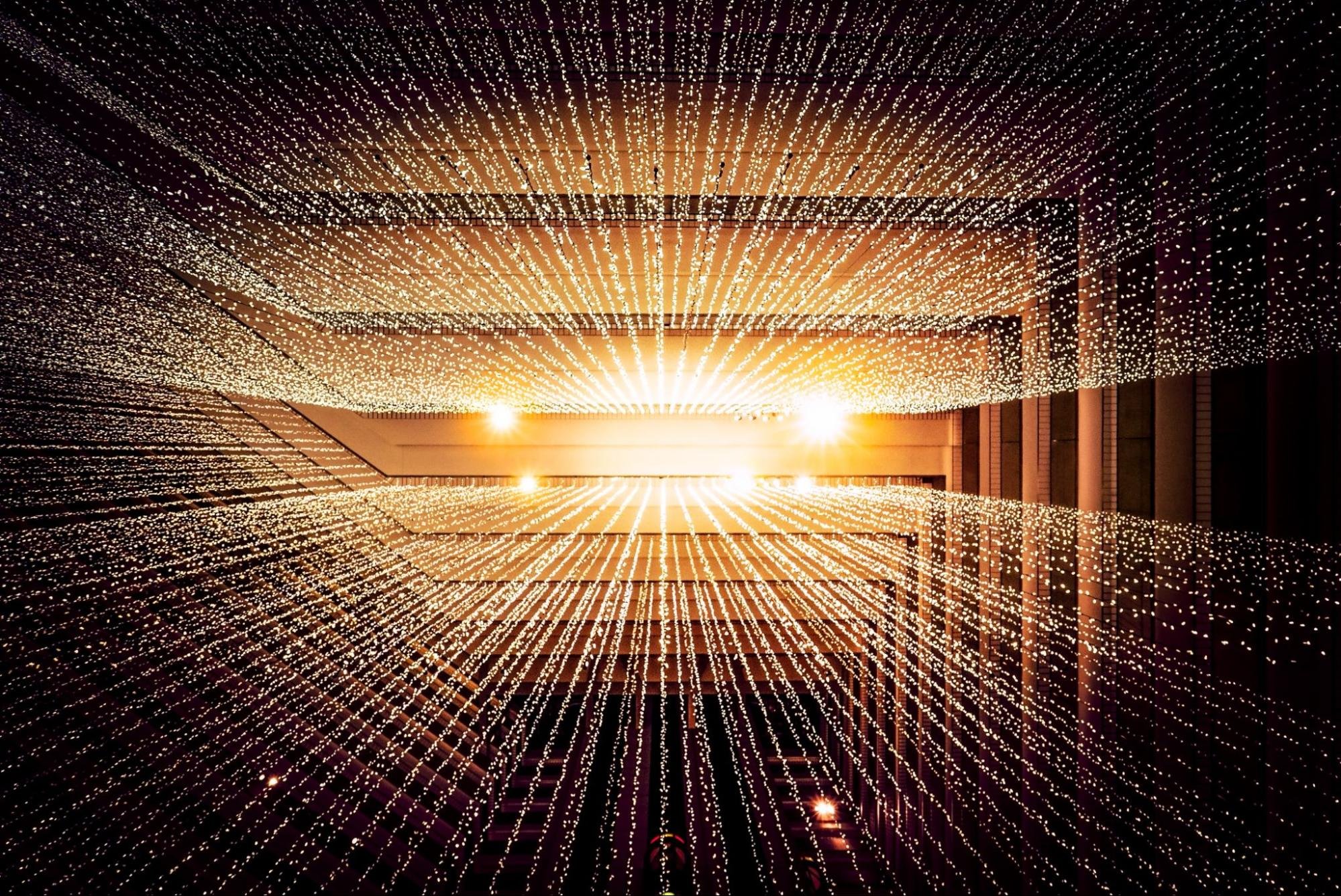